AI needs more QA, and AI QA needs more Data Science
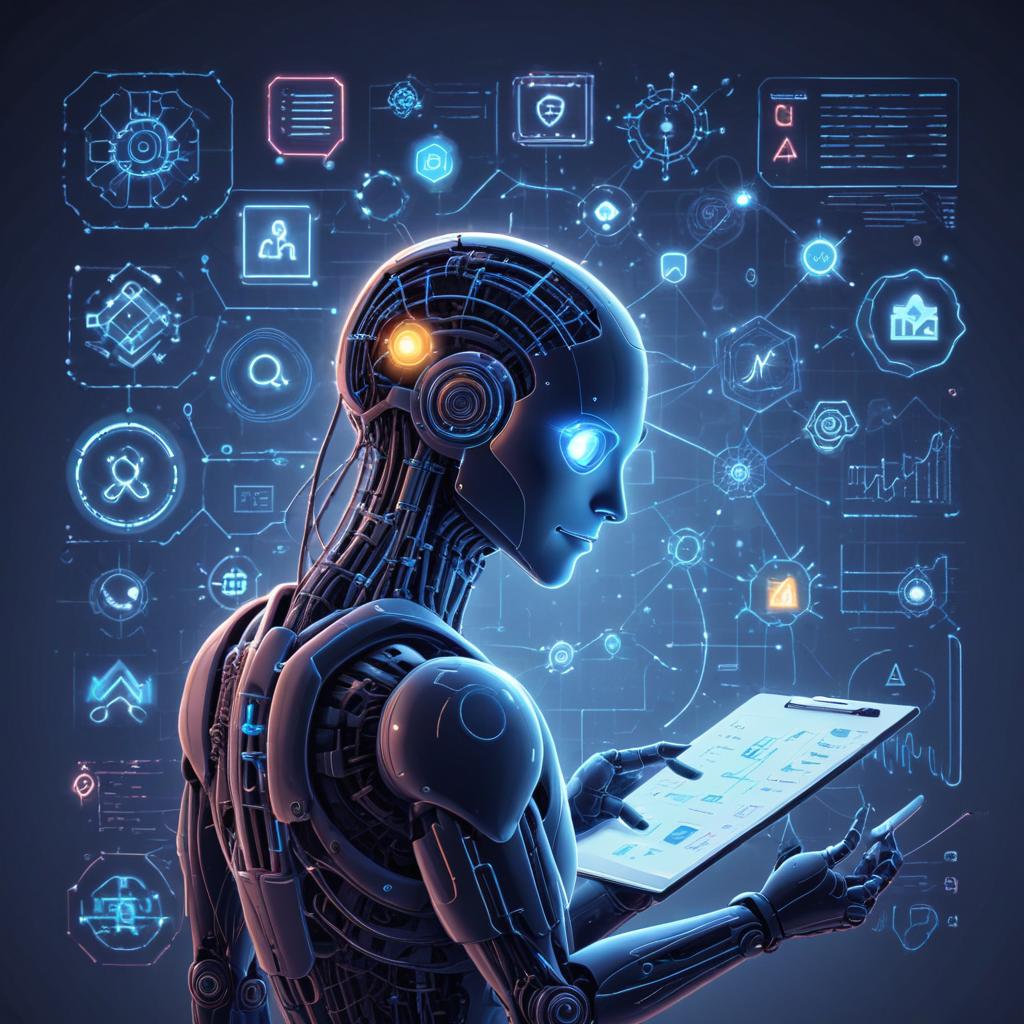
The rise of artificial intelligence (AI) has brought about new challenges and opportunities for quality assurance (QA) engineers. As AI systems become more complex and prevalent, the need for rigorous testing and validation has never been greater. At the same time, the field of AI QA is evolving rapidly, with data science playing an increasingly important role.
AI needs more QA
AI systems are complex and can exhibit unexpected behaviors, especially when deployed in real-world scenarios. Traditional software testing methods are often insufficient for ensuring the reliability and safety of AI systems. QA engineers need to develop new techniques and tools to address the unique challenges posed by AI.
Some key areas where AI needs more QA include:
- Bias and fairness: AI systems can perpetuate and amplify societal biases if not properly tested for fairness across different demographics.
- Robustness: AI models can be vulnerable to adversarial attacks that exploit weaknesses in the training data or model architecture.
- Interpretatability: It’s important to be able to understand how an AI system arrives at its decisions, especially in high-stakes domains like healthcare or finance. Scalability: As AI systems are deployed at scale, it’s critical to ensure consistent performance and reliability.
AI QA needs more Data Science
To effectively test and validate AI systems, QA engineers need to leverage data science techniques and tools. Data science can help QA engineers gain deeper insights into the behavior of AI systems and identify potential issues more effectively.
Some ways that data science can enhance AI QA include:
- Exploratory data analysis: Using data visualization and statistical techniques to understand the characteristics of the training data and identify potential issues.
- Model evaluation: Developing metrics and techniques to assess the performance of AI models on a range of tasks and datasets.
- Anomaly detection: Using machine learning algorithms to identify unusual or unexpected behaviors in AI systems.
- Predictive maintenance: Building models to predict when an AI system may experience performance degradation or failure, allowing for proactive maintenance.
The Future of AI QA
As AI continues to advance, the role of QA engineers will become increasingly important. QA engineers will need to work closely with data scientists and machine learning engineers to ensure the reliability and safety of AI systems.
Some key trends in the future of AI QA include:
- Automated testing: Using AI and machine learning techniques to automate the testing process and reduce the burden on QA engineers.
- Continuous testing: Integrating testing into the development lifecycle to ensure that AI systems are continuously validated as they evolve.
- Explainable AI: Developing techniques to make AI systems more interpretable and transparent, allowing for better understanding and trust.
- Ethical AI: Ensuring that AI systems are developed and deployed in an ethical and responsible manner, with a focus on fairness, transparency, and accountability.
In conclusion, the rise of AI has created new challenges and opportunities for QA engineers. By leveraging data science techniques and tools, QA engineers can play a critical role in ensuring the reliability and safety of AI systems. As the field of AI QA continues to evolve, it will be important for QA engineers to stay up-to-date with the latest trends and best practices.